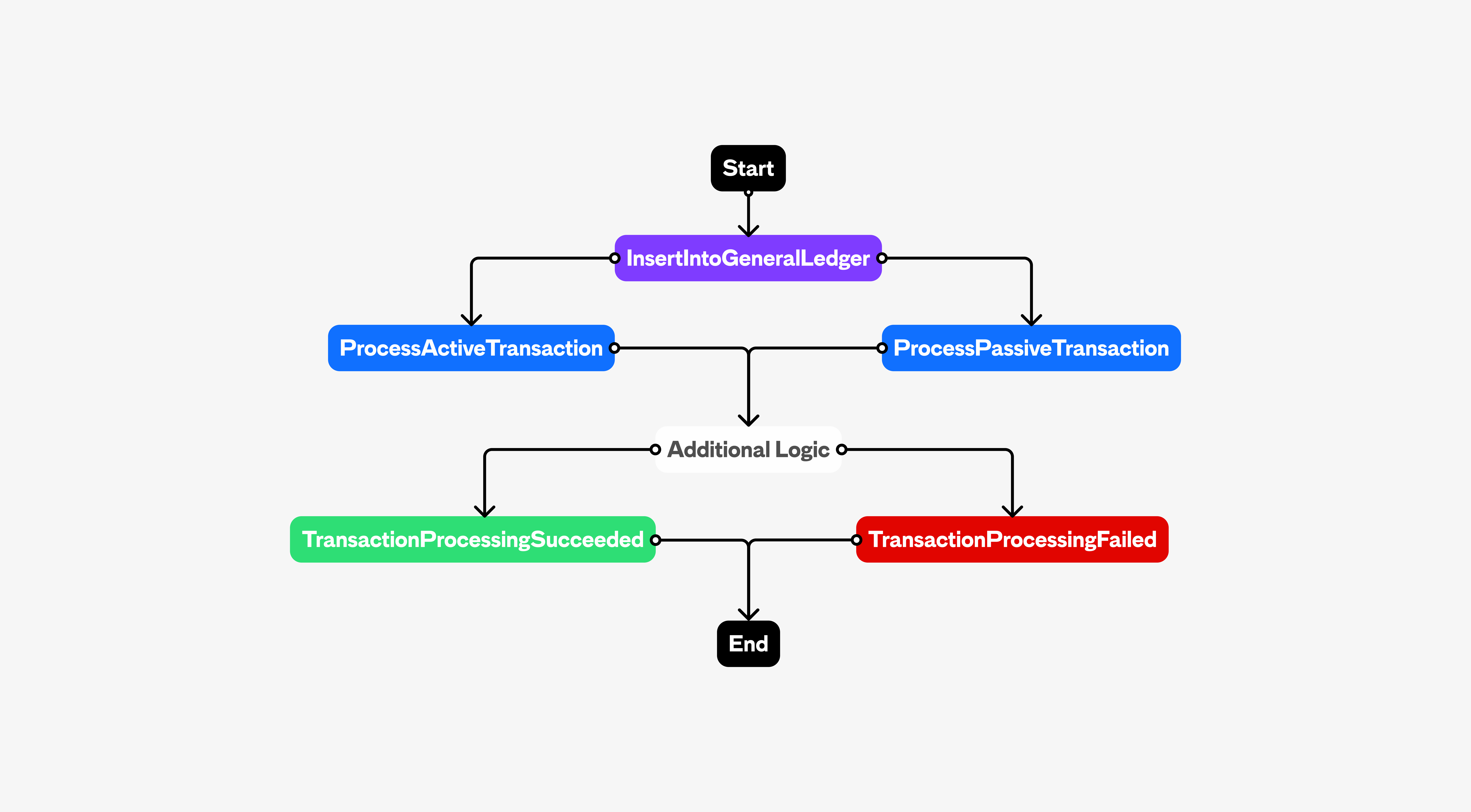
Ledgering at Ivella - Part 1
At Ivella, we’re building the best banking products for couples, and we started that journey with a new way for couples to automatically split expenses. With the Split Account, users maintain individual balances but are able to automatically split transactions by a pre-set ratio. At first glance, this problem may seem relatively straightforward (e.g. a $100.00 transaction comes in, and you charge two users $50.00). However, the reality is that there is much more complexity abstracted away from the user.
Consider a set of edge cases involved in splitting transactions: what happens when the amount changes between authorization and settlement? What happens when one party doesn’t have the balance to support their end of the transaction? What happens when a split transaction is reversed or refunded? What happens if an authorization is canceled after it has been split? Furthermore, how do you accomplish all of this without using some sort of pooled joint-custody account?
Because of this, and in order to ensure the validity of our money movement processes, one of the most critical tools we developed was our internal financial ledger. While our team is relatively new to payments, we borrowed from a long history of accounting principles and resources that provided the foundation for building our ledger. The goal of this series is to hopefully provide some insight into the intersection between accounting and software and highlight some of the processes we took to build out an auditable, maintainable, and scalable ledger.
Terminology
Before we dive into the content of this post, it is important to first lay out some of the terminology that will be used.
Transaction - A Transaction is any event coming in or out of Ivella’s system. Some examples of Transaction events that we process include purchases (e.g. a user purchasing a coffee at Starbucks), ACH originations (e.g. a user depositing money from their external account), card reversals (e.g. a user returning a purchase), etc. Transactions are grouped under two categories: Active or Passive. Active Transactions are events which are split (e.g purchases, card reversals), while Passive Transactions are events that are not split (e.g ACH originations). There are many more event types, but all are examples of Transactions.
Double Entry Accounting - DEA is a form of accounting in which all Transactions are recorded twice in a ledger. The main principle behind DEA is that each transaction consists of a credit and debit pair. For example, consider a $50.00 purchase event at Trader Joe’s by John Doe. The first entry of the ledger would reflect a $50.00 debit for John, while the second entry of the ledger would reflect a $50.00 credit for Trader Joe’s. We can represent these events on a T-account where one side reflects debits and the other reflects credits. In double entry accounting, both sides of the T-account must always balance to 0. The previous T-account may look something like this:
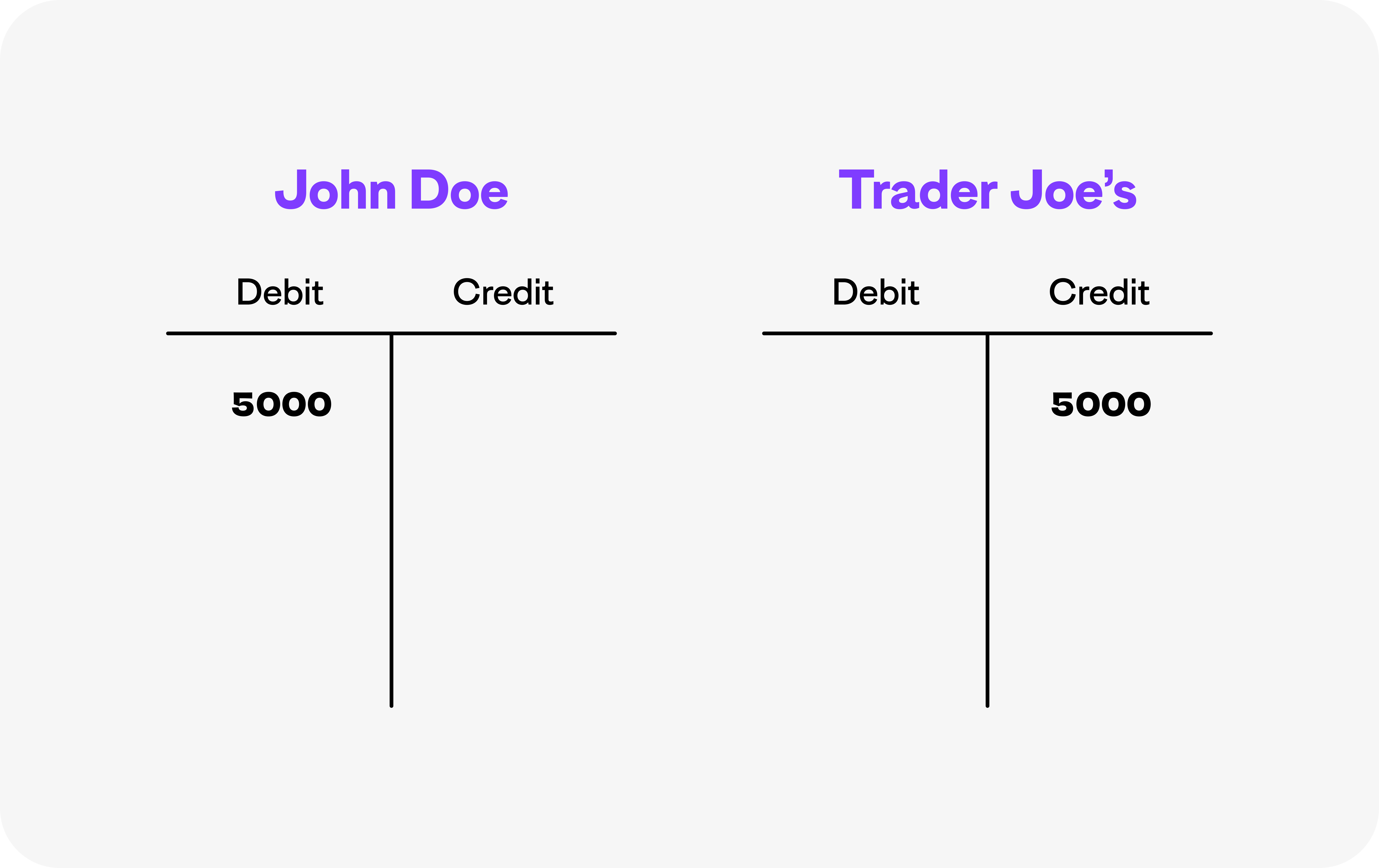
Problem
Now that we've described some of the terminology, we can discuss the main problems we wanted to address when building our ledger. Generally speaking, these problems can be grouped into three buckets.
- In order to ensure that money is moving in its intended behavior, we needed to build an immutable and auditable ledgering system that tracks all events coming in and out of our system.
- In order to handle different Transaction states, we needed to be able to track modifications to the state of any given Transaction (e.g. a purchase at Trader Joe’s goes from authorized to settled, and the corresponding debit goes from $50.00 to $72.96).
- In order to support queries for our end-users, we needed to be able to provide low latency reads and design a system that is highly scalable.
Solution
An early misconception we had while building our ledger was considering using only a few relational tables. However, we quickly realized that it was more advantageous to create multiple tables that each served a particular purpose. In order to demonstrate this, consider the previous example of a purchase Transaction at Trader Joe’s by John Doe.
When a Transaction event reaches our system, it is processed independently and carried out in a series of steps configured via AWS Step Functions. The use of AWS Step Functions not only allows us to easily orchestrate and branch our transaction processing logic, but also allows us to break apart our code into reusable microservices that can be used in various areas of the business.
The first step of the Step Function records which accounts were debited and which accounts were credited. When the aforementioned $50.00 Trader Joe’s purchase reaches the first step, we need to record both a credit entry under Trader Joe’s merchant account and a debit entry under John Doe’s Ivella account. Additionally, we need to keep track of John Doe’s balance in their Ivella Account, both before and after the purchase. To address these requirements, and as well as problem one, we created two tables: a General Ledger (GL) and an Account Subledger (AS). The GL is an immutable table that tracks every event passing through our system using Double Entry Accounting; the General Ledger serves as a single source of truth for all Transactions. Additionally, the AS is a table that tracks a user’s available and current balance, and how different Transaction events affect a user’s balance over time.
After a Transaction gets ledgered in the GL and AS, the next step of the Step Function is to ledger the Transaction on a more specific and individualized basis. For cases where a transaction’s state can be mutated, we created Event-Specific Ledgers that track state-changes for every event of a given type. For example, consider the scenario in which the purchase event at Trader Joe’s has settled and the corresponding debit changes from $50.00 to $72.96. For this scenario, the table would have one row describing the original authorization of $50.00 and another row describing the settled transaction of $72.96. All purchases and its changes are ledgered in the Purchase Events Ledger, while all ATM events and its changes are ledgered in the ATM Events Ledger. The use of Event-Specific Ledgers allows us to address problem two by keeping track of a Transaction’s lifecycle.
The Step Function then inserts an entry into the Users Transactions table. In order to address problem three, we needed to design a table specifically intended for heavy read operations. Thus, the Users Transactions table, which is configured via AWS Aurora, stores the most up-to-date information for a specific transaction. In Trader Joe's example, there would be only one row for the transaction and we would simply update that row to contain the most recent information. As opposed to the GL and Event-Specific-Ledgers, which are immutable and track state-changes via additional rows, the rows in the Users Transactions are modified to keep the table relatively small in size.
To recap, in order to correctly ledger the purchase Transaction at Trader Joes, a series of steps is carried out through the use of AWS Step Functions:
- Insertion into the General Ledger using DEA and insertion into the Account Subledger
- Insertion into the Event-Specific Ledgers to track state changes for Transactions
- Insertion into the Users Transactions table for our end users
Below is an image of an abstracted and generalized view of our Transaction Processing Step Function. There are additional steps involved depending on the specific workflow and edge case (e.g. Authorization Falloffs, ACH Returns, etc); however, for this post we will not describe those scenarios.
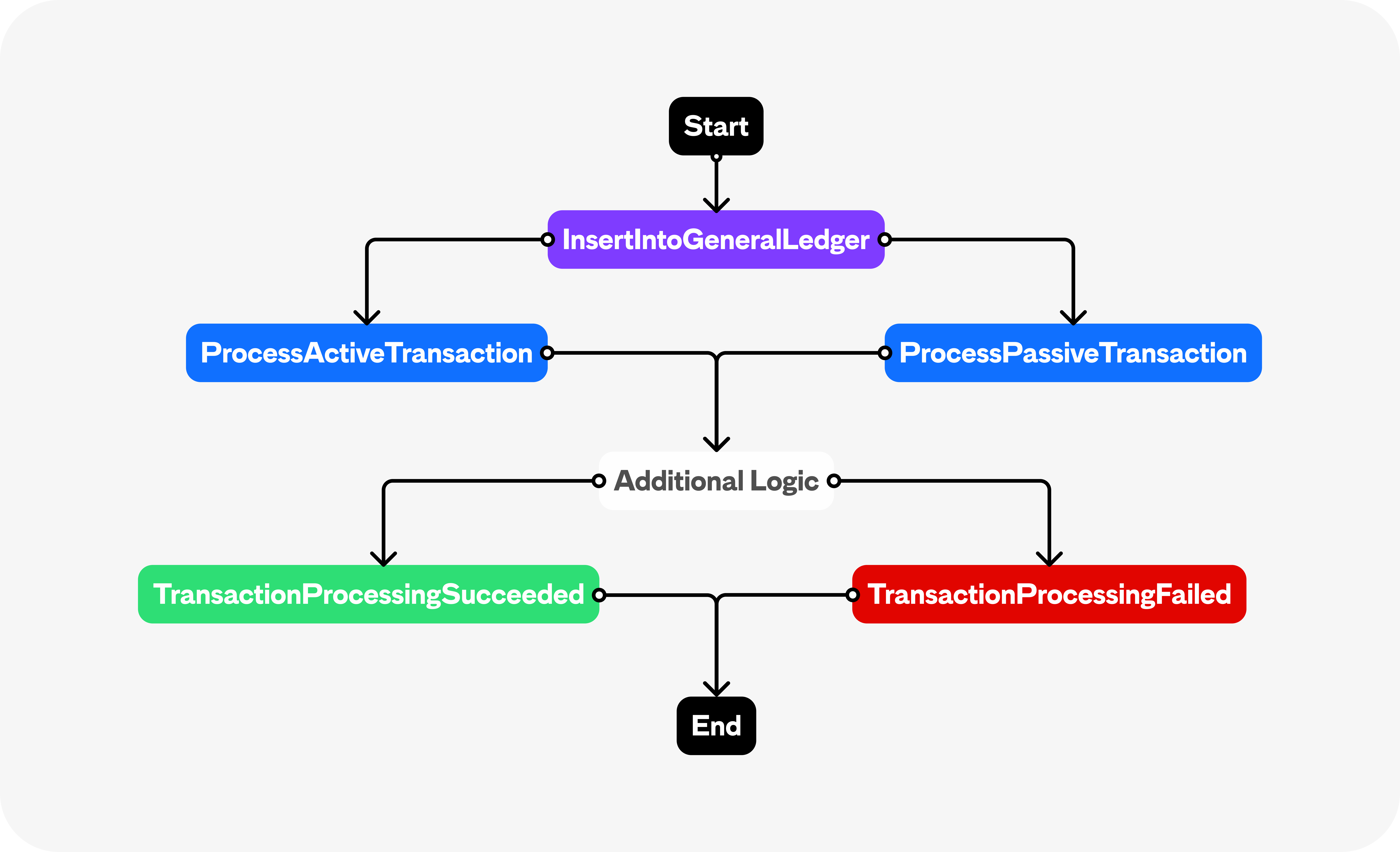
Summary
The ledgering system we created has allowed us to automate and handle the multitude of edge cases involved in splitting transactions, while ensuring the correctness of our money movement processes. Through relying on accounting principles and by creating multiple relational tables that each serve a specific purpose, we are now able to maintain an immutable and auditable ledger, track individual state changes for each Transaction, and provide low-latency queries for our end-users. We are still consistently learning and iterating on our ledger, but we hope this has provided a little insight into how we think about financial engineering problems at Ivella. In the next article, we will be doing a deeper dive into transactions subledgering from a relationship database perspective!
In the meanwhile, if you’re interested in building software solutions to payment-related problems like these, please email us here or apply here!
Hi, I’m Eric Jubber, and I recently decided to leave my software engineering job at Amazon and join Ivella. I’d like to tell you a little about what led me to make this decision, and why, after only a couple of months, I know that it was the right one.
Hi, I’m Eric Jubber, and I recently decided to leave my software engineering job at Amazon and join Ivella. I’d like to tell you a little about what led me to make this decision, and why, after only a couple of months, I know that it was the right one.
- List-1
- List-1
- List-1
- List-1
- List-1
- List-1
-
Heading-2
Heading-3
Heading-4
Heading-5
Heading-6
I worked at Amazon for just over a year, helping build the Trade-In platform. During my time there, I learned from some very experienced people (both engineers and non-engineers) and tackled problems that affected millions of customers. For an engineer looking to understand how big companies operate, Amazon was a great place to work.
After leading a few projects, I felt like I was ready for something new - something smaller, where I could have a bigger impact and learn/grow quickly.
What I Was Looking For
Amazon lives on one side of the development spectrum - hundreds of teams, thousands of engineers, and hundreds of billions in annual revenue. I knew I wanted to move to the opposite side of the spectrum. Still being early in my career, I thought that the best way to learn and grow would be to do something completely different.
A few criteria I had when considering this new role were:
• Fewer than 10 people at the company
• A strong engineering team that was working with a modern tech stack
• A role where I could help make key product and engineering decisions
• And, most of all, a company developing a product that solved a real problem
With these criteria in mind, the set of possible companies was reduced. At the same time, there were so many small teams building exciting, new products - how would I find one that was a mutual fit?
Why Ivella
Ivella stood out to me for one main reason: Ivella was solving a problem that I, along with dozens of my friends, consistently experienced: managing finances and splitting expenses in a relationship. I realized there was no great product to help partners in a relationship manage their finances, especially for those couples who are not ready to open a shared bank account.
Interviewing with Ivella only made me more excited about the opportunity. While the team was working on its first product, the Split Account, they had a suite of products in mind to decrease financial friction in relationships. Each of these products was associated with a specific problem associated with couples’ finances.
Ivella checked off the most important criterion on my list: building a product that solves a problem I experienced. What about my other criteria?
With only two engineers on the team (me joining as the third), I knew I would have the opportunities to build entirely new products, help make key decisions, and create the foundation of what could become a large, complex architecture in the future. I wanted to architect a product from the ground up, and I’d have the ability to do so at Ivella.
The team worked hard, got along well, and thought carefully about the details. During my work trial, we alternated between hours of focused work and impromptu, long product discussions that sprang out of nowhere. I loved being able to write thousands of lines of code and then debate and discuss whether partners in a relationship should earn points together or individually. Though many of our questions led to more questions, I could tell the team thought critically about everything - every assumption was challenged and no suggestion was out of the question. Outside of work, the work trial was a blast. We played Catan (our board game of choice), ate some great food in San Diego, and I got to know the team really well.
Tech-wise, the Split Account is a mobile-first banking product built with Swift/SwiftUI, GraphQL, and Node.js, deployed and scaled with a serverless infrastructure on AWS. The stack is modern and very fun to work with. The engineering culture struck a nice balance between rapidly iterating and designing with scale in mind. I was most excited to learn more about iOS/Swift and build out the GraphQL endpoints and infrastructure.
Beyond the product and tech stack, Ivella felt like a great fit. When I told them that I was only interested in joining if I could make key product and engineering decisions, they told me that that was a requirement.
My First Few Months
So, a few months into my journey with Ivella, how is everything going? It couldn't be better - joining Ivella was the right decision. Working at Ivella, or any early-stage startup, is a lot of work - but it is a different type of work from what I was used to before. There is a strong sense of ownership and a shared set of goals that we’re all constantly thinking about and working towards.
In my first few months, I’ve done a whole lot. I’ve rebuilt a number of core workflows with SwiftUI (registration, login, connect to partner, activate new card, etc…), deployed a dozen Lambdas related to card activity and transaction processing, built out a scalable in-app notifications system, written integration tests, and thought about how we are going to build a scalable and enticing points/rewards system. I’ve loved deepening my knowledge of card and transaction processing, making a number of high-level engineering decisions, and getting ready to onboard our first users.
I’m very satisfied with my experience so far and I’m excited for the future of our company. Although I’m currently working remotely, I recently got back from an awesome off-site in Palm Springs with the team, and am looking forward to heading back to Santa Monica in a few weeks and to our next off-site in Yosemite later in the Spring!
If you’re looking for a new role and anything I wrote resonated with you, please feel free to email me at ejubber@ivella.com. We’re currently hiring across engineering, product design, and marketing, and I would love to tell you more about my experience at Ivella!